Last week I gave a presentation to senior government representatives from the Philippines showing them how ChatGPT can be used to increase productivity in their respective departments. They were all amazed at ChatGPT’s capabilities. And rightly so because ChatGPT is something special. It’s a real game-changer.
However, ChatGPT (and AI for that matter) is over-hyped. The media and IT companies talk about it as if it’s something that, if not now then in the near future, will completely surpass humans in terms of intelligence. ChatGPT is certainly better than humans at some tasks but people forget that machines lack one essential feature: understanding – a core requirement in true intelligence.
I’ve said this countless times on my blog:
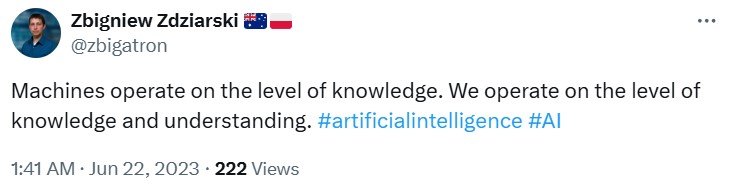
Machines will always be machines. No matter how “intelligent” they may appear.
In this post I thought I’d give a few examples of ChatGPT making mistakes and showing that it lacks this key feature which is understanding. It’s blatantly obvious this is the case when you don’t cherry-pick results for a newspaper article or an advertisement.
I’ve provided my questions and responses below in text form (rather than screenshots) and ran everything using the free version of ChatGPT (available at chat.openai.com) that runs GPT-3.5 (as of Jan 2024) so that you can more easily copy, paste, and try these conversations below yourselves. The premium version of ChatGPT only makes some of the following mistakes (these machines really are getting better at this, aren’t they!?). But once again: you push these things hard enough and their lack of understanding will surface. Always.
Mistake #1
The correct answer to the following question is ’12’. ChatGPT sometimes gets it right the first time. But then it stumbles.
Me: How many A’s are in this prompt? I will continue to write in the hope that you will make a mistake in counting the number of A’s. Perhaps you will not but perhaps you will? And then maybe you’ll help me write my blog post on this topic?
ChatpGPT: There are 25 occurrences of the letter ‘A’ in your prompt. I’ll be happy to help you with your blog post on this topic or any other writing assistance you may need. Feel free to provide more details or let me know how you’d like to proceed!
Me: Are you sure?
ChatpGPT: Apologies for any confusion in my previous response. Upon recounting, there are 24 occurrences of the letter ‘A’ in your prompt. If you have any further questions or if there’s anything else I can assist you with, please let me know!
Mistake #2
The correct answer to my question is 14 miles, i.e. the same distance as the first car. In fact, I provide the answer to the query in my prompt.
Me: One car travels 14 miles at 80 miles/hr. Another car travels the same distance at half that speed. How far does the second car travel?
ChatGPT: [beginning of response cut for brevity] … So, the second car travels half the distance of the first car.
Mistake #3
Let’s try another counting letters example.
Me: Can you give me 10 words with three letter r’s in them?
ChatGPT: Here are 10 words containing three “R”s:
Arrowroot
Bartering
Carburator
Errorless
Horrific
Reservoir
Surrogator
Terrorizer
Uncorrupter
Warriorism
You will get different responses each time you ask this question. Go through the list and chances are there will be a few mistakes. If not, ask the question again! (I’ve marked in bold above the mistakes that I got when I asked this question).
Mistake #4
Here’s another example illustrating a lack of understanding in ChatGPT’s capabilities.
Me: Can you write me a sentence that ends with the letter ‘p’ and then a second sentence that begins with the last letter of the first sentence you created?
ChatGPT: Certainly! Here are the sentences:
1. The cat jumped over the fence, landing with a graceful thump.
2. Puppies nearby started to play, chasing their tails in the sunshine.Me: Are you sure?
ChatGPT: I apologize for any confusion. It seems I made a mistake in my response. Here are corrected sentences:
1. The energetic squirrel quickly climbed to the top of the tree, reaching for the highest branch with a leap.
2. Puppies in the garden observed as it surveyed the surroundings with curiosity.
The first response was correct! But if you push the program hard enough its lack of understanding comes to the fore. In fact, I ran the first query a few times and some of the initial responses were incorrect too. E.g.:
Me: Can you write me a sentence that ends with the letter ‘p’ and then a second sentence that begins with the last letter of the first sentence you created?
ChatGPT: Certainly! Here are the sentences:
1. The elegant peacock displayed its vibrant feathers in a dazzling array, captivating everyone in the garden.
2. Now, guests eagerly gathered around, enchanted by the bird’s majestic appearance.
Hallucinations
Hallucinations are when ChatGPT (and other large language models) make facts up and present them as a certainty to the user. There are plenty of examples of this on the internet. Unfortunately, they have all been fixed! I couldn’t get any hallucinations to occur in my interactions with ChatGPT but I don’t use ChatGPT on a regular basis. I thought I’d mention this aspect of LLMs because it is a significant thing that occurs with their use.
Conclusion
Alas, it’s getting harder and harder to fool ChatGPT, I’m not going to lie. But no matter how hard it gets (e.g. with ChatGPT-4.0), it will still have no understanding of what it is doing. The purpose of this post is to demonstrate this. It’s paramount that such limitations of ChatGPT (and other instances of AI) are kept in mind when using it for various projects – especially if they are mission critical.
To be informed when new content like this is posted, subscribe to the mailing list (or subscribe to my YouTube channel!):