This is the 50th post on my blog. Golden anniversary, perhaps? Maybe not. To celebrate this milestone, however, I thought I’d return to my very first post that I made at the end of 2017 (4 years ago!) on the topic of image enhancing scenes in Hollywood films. We all know what scenes I’m talking about here: we see some IT expert scanning security footage and zooming in on a face or a vehicle licence plate; when the image becomes blurry the detective standing over the expert’s shoulder requests for the image to be enhanced. The IT guy waves his wand and presto!, we see a full resolution image on the screen.
In that previous post of mine I stated that, although what Hollywood shows is rubbish, there are actually some scenarios where image enhancing like this is possible. In fact, we see it in action in some online tools that you may even use every day – e.g. Google Maps.
In today’s post, I wish to talk about new technology that has recently emerged from Google that’s related to the image enhancing topic discussed in my very first post. The technology I wish to present to you, entitled “High Fidelity Image Generation Using Diffusion Models“, was published on the Google AI Blog in July of this year and is on the topic of super-resolution imaging. That is, the task of transforming low-resolution images into detailed high resolution images.
The difference between image enhancing (as discussed in my first post) and super-resolution imaging is that the former gives you faithful, high-resolution representations of the original object, face, or scene, whereas the latter generates high-resolution images that look real but may not be 100% authentic to the original scene of which the low-resolution image was a photograph. In other words, while super-resolution imaging can increase the information content of an image, there is no guarantee that the upscaled features in the image exist in the original scene. Hence, the technique should be used with caution by law enforcement agencies for things like enhancing images of faces or licence plate numbers!
Despite this, super-resolution imaging has its uses too – especially since the generated images can be quite similar to the original low-resolution photo/image. Some applications include things like restoring old family photos, improving medical imaging systems, and the simple but much desired need of deblurring of images.
Google’s product is a fascinating one, if not for the fact that its results are amazing. Interestingly, the technology behind the research is not based on deep generative models such as GANs (Generative Adversarial Networks – I talk about these briefly in this post), as one would usually expect for this kind of use case. Google decided to experiment with diffusion models, which is an idea first published in 2015 but much neglected since then.
Diffusion models are very interesting in the way they train their neural networks. The idea is to first progressively corrupt training data by adding Gaussian noise to it. Then, a deep learning model is trained to reverse this corruption process with reference to the original training data. A model trained in this way is perfect for the task of “denoising” lower resolution images into higher ones.
Let’s take a look at some of the results produced by this process and presented by Google to the world:
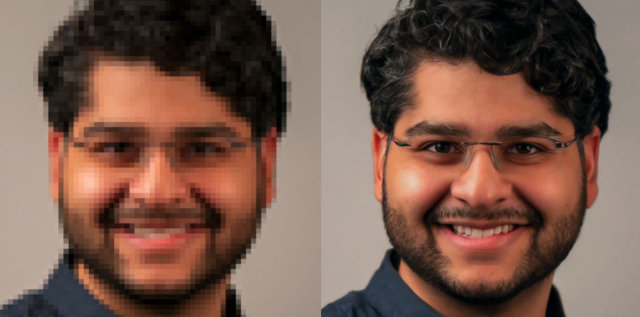
That’s pretty impressive considering that no additional information is given to the system about how the super-resolutioned image should look. The result looks like a faithful up-scaling of the original image. Here’s another example:
Google reports that its results far surpass those of previous state-of-the-art solutions for super-resolution imaging. Very impressive.
But there’s more. The researchers behind this work tried out another interesting idea. If one can get impressive results in upscaling of images as shown above, how about taking things a step further and chaining together multiple models trained at upscaling at different resolutions. What this has produced is a cascading effect of upscaling that can create higher resolution images from mere thumbnails of images. Have a look at some of these results:
It’s very impressive at how these programs can “fill-in the blanks”, so to speak, and create more details in an image when it’s needed. Although some results aren’t always accurate (images may have errors in them like discontinuities or gaps where none should appear), but generally speaking, these upscaled images would pass off as genuine at first glance by most users.
Google has undoubtedly struck again.
That’s it for my 50th post. It’s been a great ride so far on this blog. Hopefully there’s lots more to come. Tell your friends 🙂
To be informed when new content like this is posted, subscribe to the mailing list: