(Update: part 3 of this post was posted recently here.)
In July of last year I wrote an opinion piece entitled “Artificial Intelligence is Slowing Down” in which I shared my judgement that as AI and Deep Learning (DL) currently stand, their growth is slowly becoming unsustainable. The main reason for this is that training costs are starting to go through the roof the more DL models are scaled up in size to accommodate more and more complex tasks. (See my original post for a discussion on this).
In this post, part 2 of “AI Slowing Down”, I wanted to present findings from an article written a few months after mine for IEEE Spectrum. The article, entitled “Deep Learning’s Diminishing Returns – The cost of improvement is becoming unsustainable“, came to the same conclusions as I did (and more) regarding AI but it presented much harder facts to back its claims.
I would like to share some of these claims on my blog because they’re very good and backed up by solid empirical data.
The first thing that should be noted is that the claims presented by the authors are based on an analysis of 1,058 research papers (plus additional benchmark sources). That’s a decent dataset from which significant conclusions can be gathered (assuming the analyses were done correctly, of course, but considering the four authors who are of repute, I think it is safe to assume the veracity of their findings).
One thing the authors found was that with the increase in performance of a DL model, the computational cost increases exponentially by a factor of four (i.e. to improve performance by a factor of k, the computational cost scales by k^4). I stated in my post that the larger the model the more complex tasks it can perform, but also the more training time is required. We now have a number to estimate just how much computation power is required per improvement in performance. A factor of four is staggering.
Another thing I liked about the analysis performed was that it took into consideration the environmental impact of growing and training more complex DL models.
The following graph speaks volumes. It shows the error rate (y-axis and dots on the graph) on the famous ImageNet dataset/challenge (I’ve written about it here) decreasing over the years once DL entered the scene in 2012 and smashed previous records. The line shows the corresponding carbon-dioxide emissions accompanying training processes for these larger and larger models. A projection is then shown (dashed line) of where carbon emissions will be in the years to come assuming AI grows at its current rate (and no new steps are taken to alleviate this issue – more on this later).
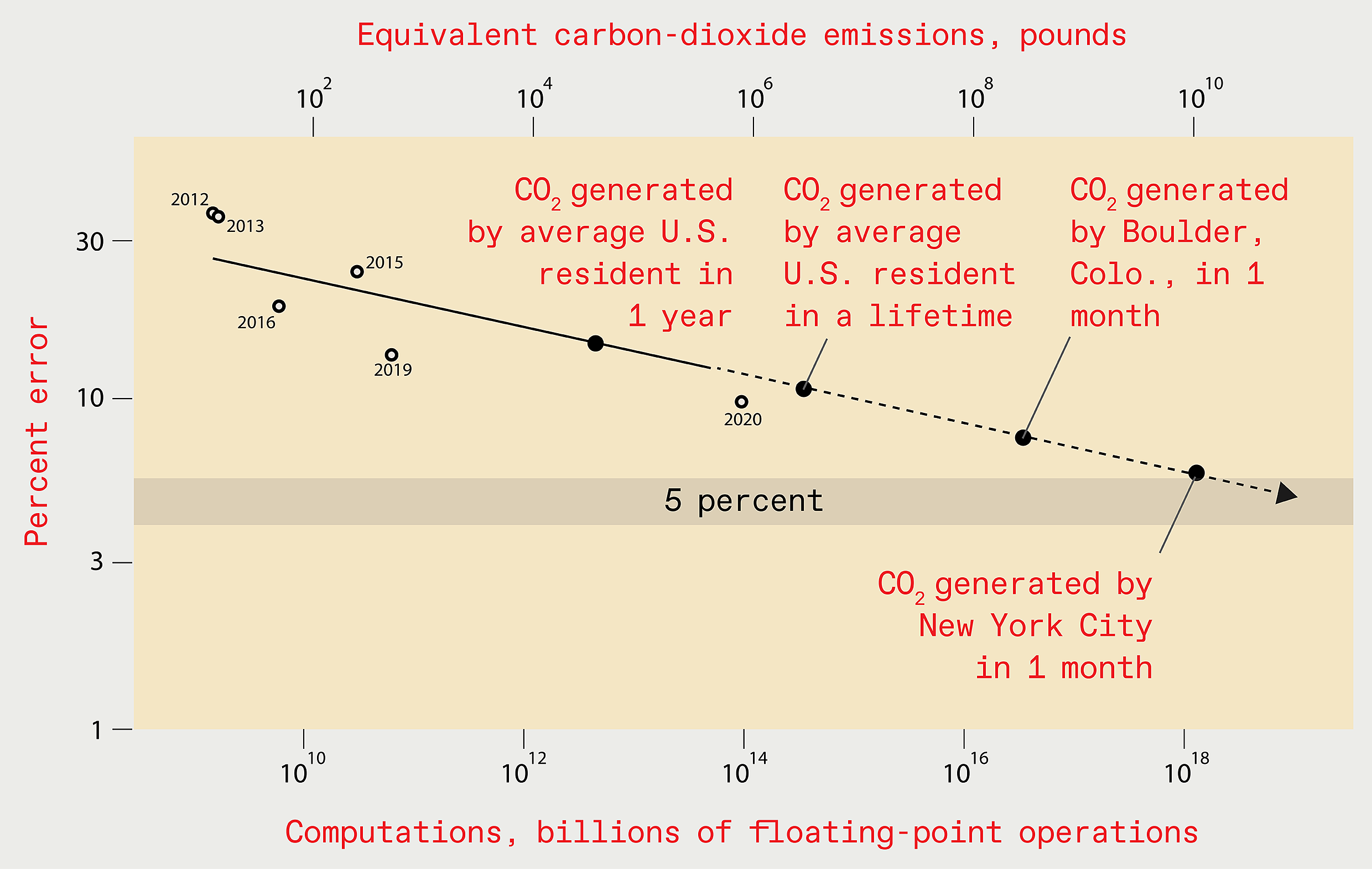
Just look at the comments in red in the graph. Very interesting.
And the costs of these future models? To achieve an error rate of 5%, the authors extrapolated a cost of US$100 billion. That’s just ridiculous and definitely untenable.
We won’t, of course, get to a 5% error rate the way we are going (nobody has this much money) so scientists will find other ways to get there or DL results will start to plateau:
We must either adapt how we do deep learning or face a future of much slower progress
At the end of the article, then, the authors provide an insight into what is happening in this respect as science begins to realise its limitations and look for solutions. Meta-learning is one such solution that is presented and discussed (meta-learning is the training of models that are designed for broader tasks and then using them for a multitude of more specific cases. In this scenario, only one training needs to take place for multiple tasks).
However, all the current research so far indicates that the gains from these innovations are minimal. We need a much bigger breakthrough for significant results to appear.
And like I said in my previous article, big breakthroughs like this don’t come willy-nilly. It’s highly likely that one will come along but when that will be is anybody’s guess. It could be next year, it could be at the end of the decade, or it could be at the end of the century.
We really could be reaching the max speed of AI – which obviously would be a shame.
Note: the authors of the aforementioned article have published a scientific paper as an arXiv preprint (available here) that digs into all these issues in even more detail.
To be informed when new content like this is posted, subscribe to the mailing list (or subscribe to my YouTube channel!):
7 Replies to “Artificial Intelligence is Slowing Down – Part 2”