Google Glass was a flop. But what about glasses for the blind that tell the wearer what is in front of them? Or read a physical book for them?
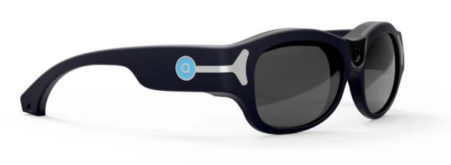
Posts discussing object detection and tracking
Google Glass was a flop. But what about glasses for the blind that tell the wearer what is in front of them? Or read a physical book for them?
In this post I present to you quite an amazing paper I stumbled upon entitled “Soccer on Your Tabletop” from CVPR 2018.
In my last two posts (part 1 can be found here, part 2 can be found here) I’ve looked at computer vision and the fashion industry. I introduced the lucrative fashion industry and showed what Microsoft recently did in this field with computer vision. I also presented two papers from last year’s International Conference on Computer […]
(image source) (Update: this post is the first of a 3-part series. Part 2 can be found here, part 3 can be found here) Computer vision has a plethora of applications in the industry: cashier-less stores, autonomous vehicles (including those loitering on Mars), security (e.g. face recognition) – the list goes on endlessly. I’ve already […]
In last week’s post I talked about plotting tracked customers or staff from video footage onto a 2D floor plan. This is an example of video analytics and data mining that can be performed on standard CCTV footage that can give you insightful information such as common movement patterns or common places of congestion at […]
Data mining is a big business. Everyone is analysing mouse clicks, mouse movements, customer purchase patterns. Such analysis has proven to give profitable insights that are driving businesses further than ever before. But not many people have considered data mining videos. What about all that security footage that has stacked up over the years? Can […]
In this post I’m going to present to you how, where, and when computer vision was used by the Mars Exploration Rovers (MERs) that landed on Mars in 2004.
Where would a computer vision blog be without a post about the new cashier-less store recently opened to the public by Amazon? Absolutely nowhere. But I don’t need additional motivation to write about Amazon Go (as the store is called) because I am, to put it simply, thrilled and excited about this new venture. This is innovation […]
I was watching The Punisher on Netflix last week and there was a scene (no spoilers, promise) in which someone was recognised from CCTV footage by the way they were walking. “Surely, that’s another example of Hollywood BS“, I thought to myself – “there’s no way that’s even remotely possible”. So, I spent the last […]